In the fast-paced world of data-driven decision-making, eCommerce brands are on a quest to build robust data infrastructure that can unlock valuable insights and provide them with a competitive edge.
Traditionally, the go-to approach has been to construct an in-house data stack, meticulously piecing together various components to form a cohesive system - but it comes with its own set of challenges and costs.
However, with the advent of content management systems (CMS) like Shopify, a revolution has occurred. CMS platforms come with structured data, which fundamentally changes the landscape of data infrastructure. In this new era, the concept of a vertical data platform becomes highly relevant.
In this blog post, we will explore the true cost of building a data stack internally for an eCommerce brand and why opting for an all-in-one platform can be a compelling alternative.

The Components of a Data Stack
A robust data stack consists of various components, each serving a specific purpose. Let's examine these components and their associated costs:
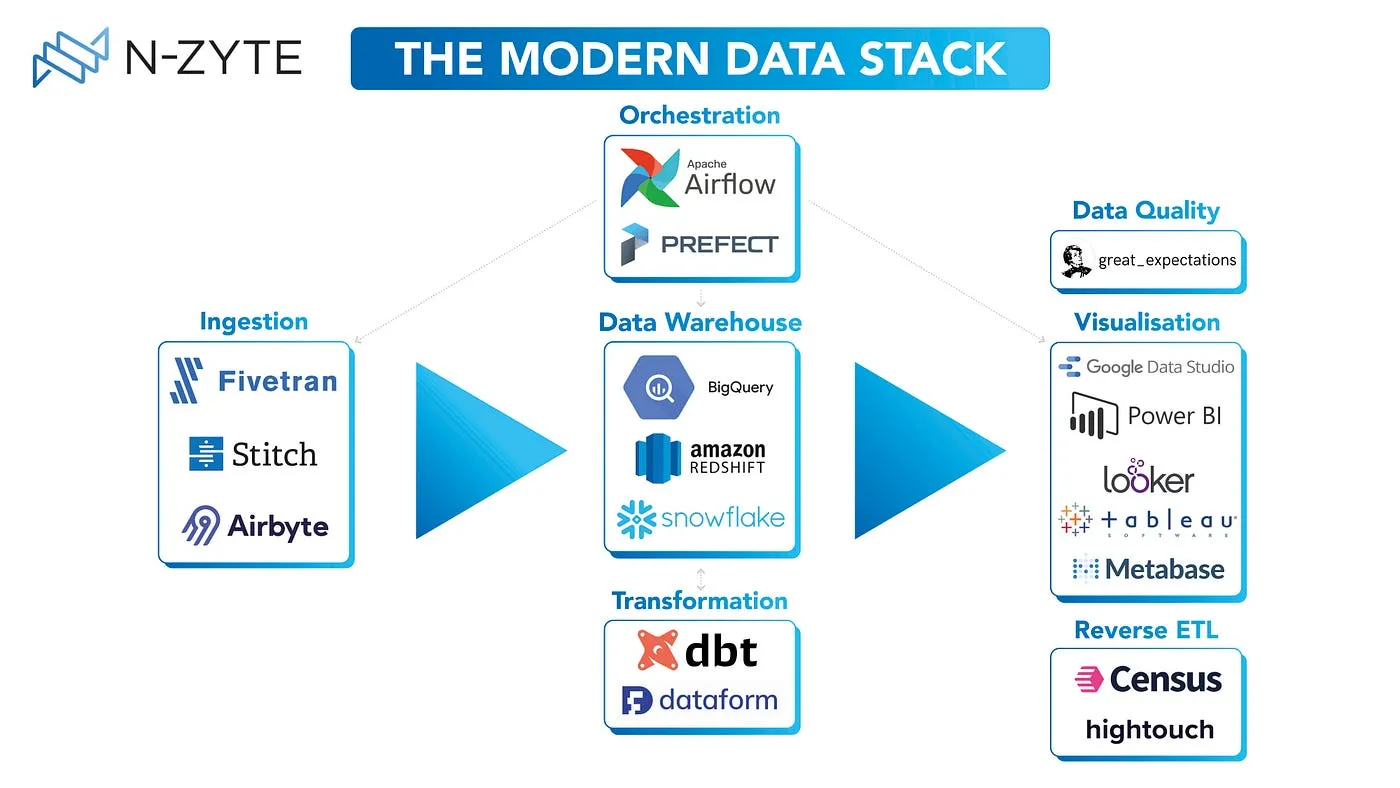
Data Integration/Pipelines:
Data integration is a critical component of a data stack as it involves consolidating data from various sources into a central location.
Tools like Fivetran and ETL solutions such as Stitch or Talend are commonly used for data integration. These tools automate the process of extracting data from different sources, transforming it into a unified format, and loading it into a data warehouse. They ensure that data is collected efficiently, accurately, and in a timely manner.
While the costs of these tools can range from $1,000 to $5,000 per month, they offer significant value by streamlining the data integration process and reducing manual effort.
Data Storage:
Once the data is collected, it needs to be stored and managed in a secure and scalable environment. Cloud data warehouses like Snowflake and BigQuery are popular choices for eCommerce brands.
These warehouses provide storage, computational power, and built-in scalability to handle large volumes of data. Snowflake's pricing typically ranges from $400 to $1,000 per month, while BigQuery may cost around $300 to $700 per month. Cloud data warehouses eliminate the need for managing on-premises infrastructure and provide flexibility in terms of data storage and processing capabilities.
Data Modelling / Business Logic Layer:
The logic layer is responsible for transforming raw data into analysis-friendly formats, enabling data analysts to derive meaningful insights.
DBT (Data Build Tool) is a widely used open-source solution for data transformation. DBT automates data modeling, schema management, and transformation processes. It allows data analysts to define business logic and create reusable SQL code, ensuring consistent data transformations across the organization.
While the open-source version of DBT is free, opting for the hosted version, DBT Cloud, offers additional features and support, with pricing ranging from $50 to $500+ per month. Implementing DBT requires setting up and configuring the tool, defining transformation models, and running the necessary commands to transform and load data.
Data Visualization:
Data visualization tools play a crucial role in turning complex data into understandable and actionable insights.
Looker and Google Data Studio are popular choices in the eCommerce industry. Looker provides advanced analytics and visualization capabilities, allowing users to create interactive dashboards, reports, and data exploration experiences. Pricing for Looker can vary, but it is estimated to start from $3,000 to $5,000 per month for a business-level package.
On the other hand, Google Data Studio offers a free version that allows users to create basic visualizations and reports using data from various sources. Implementing data visualization tools involves connecting to the data source, defining visualizations and metrics, and designing intuitive dashboards and reports.
Data Governance and Quality:
To ensure the reliability, accuracy, and trustworthiness of data, eCommerce brands require data governance, quality, and monitoring tools.
These tools help establish data standards, enforce data policies, perform data profiling, and monitor data quality. Popular tools in this category include Collibra, Alation, and Trifacta. Costs for these tools can range from $1,000 to $2,000 per month, depending on the features and capabilities offered.
Implementing data governance and quality tools involves configuring data policies, establishing data governance workflows, and setting up monitoring and alerting mechanisms.
Cost Breakdown of the Traditional Stack
Adding up the costs of each component, the total tool expenses of a traditional data stack for an eCommerce brand, factoring in salaries for data engineers and data analysts, could escalate to $300k/ to $500k+ per year. These figures highlight the significant financial investment required.

Why brands might prefer an all-in-one platform
- Cost Efficiency: Building an in-house data stack requires significant upfront investment and ongoing costs. It involves expenses related to infrastructure, tooling, licenses, maintenance, and hiring skilled personnel. On the other hand, an all-in-one platform typically offers a bundled pricing model that can be more cost-effective, especially for smaller and medium-sized eCommerce brands. It eliminates the need for separate subscriptions and reduces the total cost of ownership.
- Time to Value: Developing an in-house data stack involves a time-consuming process of researching, selecting, integrating, and maintaining multiple tools. It requires building custom pipelines, transformations, and data models, which can take months or even years to complete. In contrast, an all-in-one platform provides pre-built integrations, ready-to-use features, and streamlined workflows, enabling faster implementation and quicker time to value. Brands can focus on utilizing the data rather than spending time on infrastructure development.
- Seamless Integration: An all-in-one platform is designed to work cohesively, ensuring seamless integration between various components like data ingestion, transformation, visualization, and governance. This eliminates the need for complex integration efforts and reduces the risk of compatibility issues that may arise when piecing together individual tools in an in-house stack. The integrated nature of an all-in-one platform simplifies the overall data management process.
- Scalability and Flexibility: As eCommerce brands grow and data volumes increase, scaling an in-house data stack can be challenging. It requires additional investments in infrastructure, resource allocation, and maintaining performance. In contrast, all-in-one platforms are built to scale effortlessly, handling data growth without significant disruptions. They often provide flexible pricing plans that can adapt to changing business needs, allowing brands to expand their data capabilities without major overhauls.
- Expert Support and Updates: All-in-one platforms typically offer dedicated support from experts who specialize in their specific tools and functionalities. They provide ongoing assistance, troubleshooting, and guidance to ensure smooth operations. Additionally, these platforms regularly release updates and enhancements, keeping up with industry trends and ensuring access to the latest features and capabilities. This saves brands the effort and resources required to stay up-to-date and maintain their in-house stack.
- Focus on Core Competencies: Building and maintaining an in-house data stack requires significant time, effort, and expertise. For eCommerce brands, their core focus should be on their products, customer experience, and business growth. By leveraging an all-in-one platform, brands can offload the complexities of managing a data stack and redirect their resources and attention towards their core competencies, allowing them to maximize their business potential.
It's important to note that the decision between an all-in-one platform and an in-house data stack depends on various factors like the brand's size, budget, specific requirements, and long-term strategy.
The Cons of an All-In-One Solution
While all-in-one platforms like Polar Analytics can offer many advantages, it's crucial to acknowledge the potential downsides. Here are a few things to consider:
- Limited Customization: Since all-in-one solutions are designed to accommodate a broad range of use-cases, they might not offer the same level of customization as individual tools. For businesses with very specific or unique needs, this could be a limitation.
- Less Control: When using an all-in-one platform, the company may have less control over the details of the data infrastructure. For some businesses, this lack of control might not be ideal, especially if they have unique compliance, security, or operational needs.
In conclusion, while building an in-house data stack may be necessary for some businesses, others may find greater value in adopting an all-in-one platform.
The experience of Tiege is a clear demonstration of the struggles businesses can face when building an internal data stack and the potential benefits that can be gained by switching to a comprehensive solution like Polar Analytics.
The key is to carefully assess your business needs, resources, and strategy before making a decision.
Case Study: Tiege and the Pitfalls of an In-house Data Stack
Let's illustrate the points mentioned above with a real-life example - Tiege. Tiege is an eCommerce brand that attempted to build their own data stack, leveraging tools such as Fivetran for data integration, Snowflake for data storage, and Tableau and PowerBI for data visualization.
Their experience in constructing an in-house data stack mirrored the challenges and costs mentioned previously. Despite a hefty monthly expenditure, Tiege struggled to derive actionable insights from their data.
Even with a full-time data scientist on the team, the road to insights proved to be a long one, stretching over two years.
One of the main obstacles they faced was the technical complexity of their data stack. The tools they chose, while powerful, were too technical for their business teams in marketing, operations, and finance to effectively use. They found it challenging to move from raw data to actionable insights, creating a bottleneck in their decision-making process.
Their solution came in the form of an all-in-one platform - Polar Analytics. Switching to Polar allowed Tiege to simplify their data operations and focus on deriving insights rather than struggling with the complexities of data engineering and cleaning.
The modular nature of the app allowed Tiege to utilize their Snowflake instance and get rid of the need for Fivetran and Tableau, simplifying their data stack and reducing costs.
The supportive environment provided by Polar Analytics was also a game-changer for their data scientist. The chance to interact with other data specialists was a welcome opportunity, breaking the echo chamber effect and facilitating collaborative problem solving.

Conclusion
In conclusion, the traditional approach of building an in-house data stack for eCommerce brands involves substantial costs and effort.
However, the rise of CMS platforms and the concept of vertical data platforms present new possibilities. By adopting an all-in-one platform like Polar Analytics, eCommerce brands can benefit from cost efficiency, faster implementation, seamless integration, scalability, expert support, and the ability to focus on their core competencies.
The decision between an all-in-one platform and an in-house data stack ultimately depends on the brand's specific needs and long-term strategy. However, in the dynamic eCommerce landscape, embracing an all-in-one platform can provide a competitive advantage and open doors to exciting new opportunities.
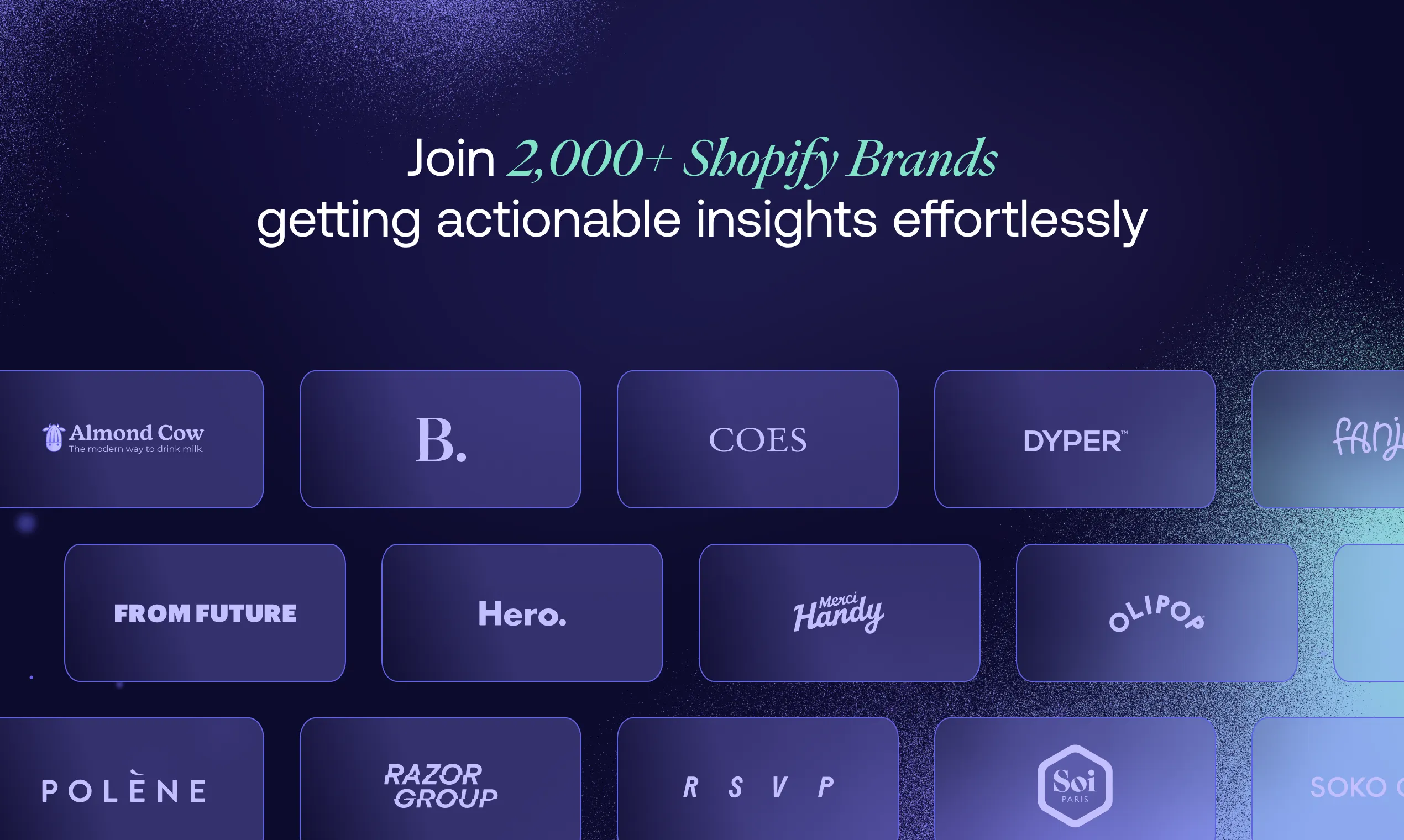